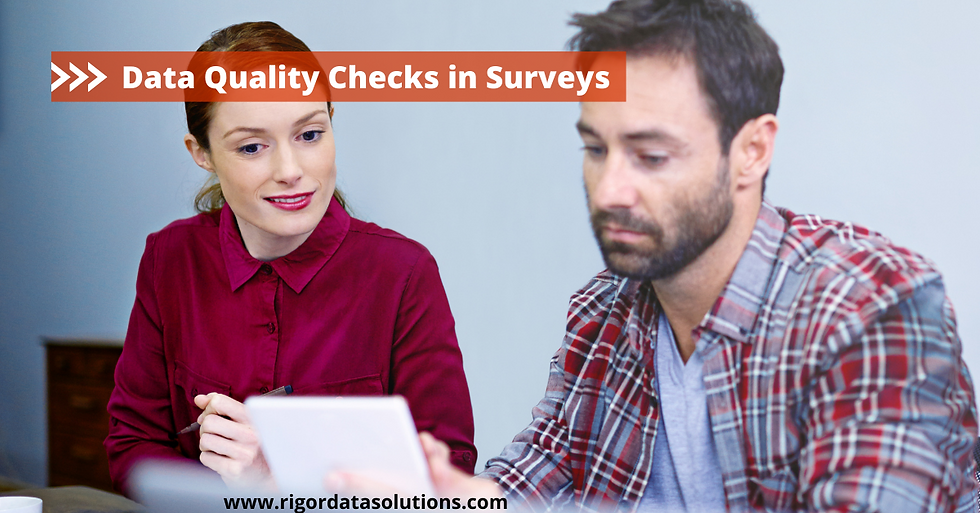
Introduction
Finally, you are through with the hefty task of planning your survey. All the requirements for your survey have been double-checked in readiness for the survey day.
You know very well the objective of the survey
You’ve determined who to participate in the survey (target population)
You’ve decided on the type of survey (mail, online, or in-person)
You’ve designed the survey questions and layout
You’ve recruited the data collection teams and trained them
You’ve planned and organized all logistical needs
You’ve got the permit required to conduct the survey etc.
In summary we can say, everything and everyone is ready to start the project. It’s all systems go!
But one last thing needs to be done. You need to set-up a system to monitor the quality of data collected. This system should track a set of data quality metrics – and automatically flag instances that indicate data fraud or data fabrication – empowering project supervisors and leadership easily identify breaches and address them before it’s too late.
In this article, I share ten data quality metrics you should measure or track, to assess and guarantee the quality of your survey data!
1. The length of the survey (time it takes to complete each survey)
This could be measured by computing the difference between interview start time and interview end time, to see the time each enumerator took to complete the survey. These metrics can be compared with the average interview time (computed from the pilot survey) to flag enumerators taking too much time, or too little time to complete the survey (which could be an indicator that the enumerator is fabricating data).
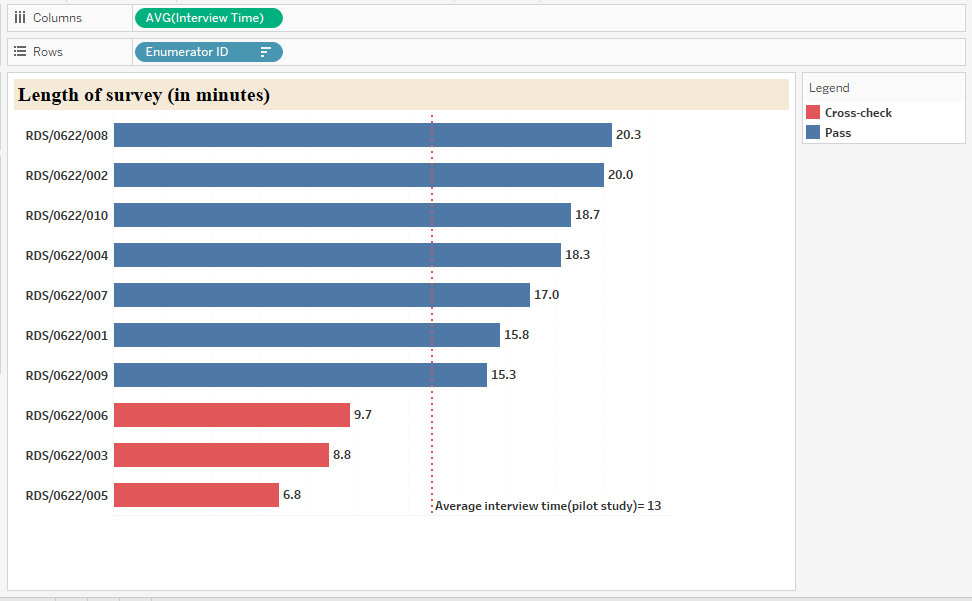
Once you have identified enumerators with anomalies – you can further break-down the analysis by interview – to highlight the individual interviews with anomalies, which you can cross-check and address.
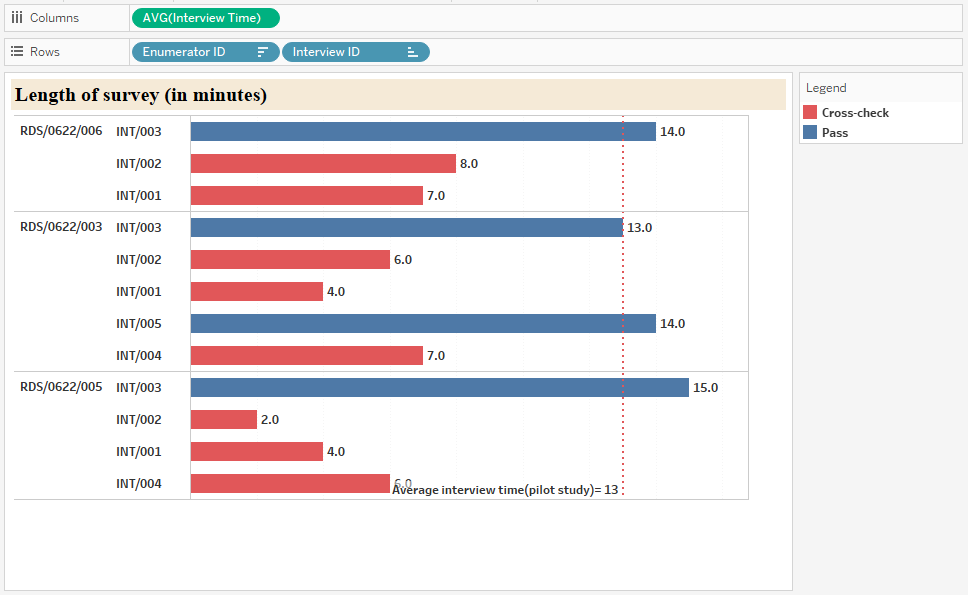
2. Number of inconsistent responses
This can be simple as checking enumerators with high number of impossible responses (demographic inconsistencies)
For example,
A case whereby the head of household is 28 years with 15 children.
A case whereby the respondent’s education level is college, but in other question is captured as illiterate.
A case whereby the respondent has been employed for 25 years or is recorded as retired, when their actual is below 25 years.
More related cases can easily be identified and tracked to flag enumerators fabricating data.
3. Location audit using GPS
Depending on whether your devices can record the GPS location of the interviewers, you can use such data to see if an enumerator is where they are supposed to be, or they are staying in one place and completing multiple surveys – which can signify data fraud.
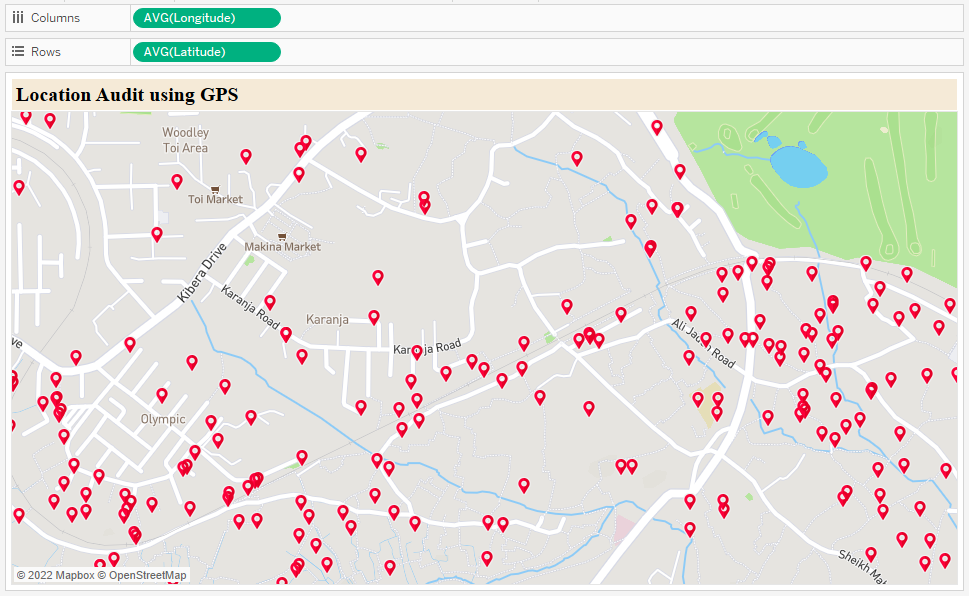
(Sample Image showing the GPS locations of where the interviews took place)
4. Number of ‘No’ responses for skip orders
For questions which trigger additional questions when a respondent answers ‘Yes’ might fraudulently be reported as ‘No’ so that the enumerator can do less work. This can be checked by comparing the rate of ‘No’ responses across enumerators.
(Rate of No responses = Number of ‘No’ responses for questions which trigger additional questions when the respondent’s answer is ‘Yes’/Total number of questions which trigger additional questions when the respondent’s answer is ‘Yes’)

Enumerators with higher rate of ‘No’ responses for skip orders could further be cross-checked, or closely monitored for data fraud.
5. Track quality check questions
In cases whereby the survey includes quality check questions – quality check questions are questions which ask the respondent to select a specific item.
For such cases you can track these quality check questions to flag instances where the responses deviate from the expectations (which could indicate inattentiveness or data fraud).
6. Number of surveys completed per day
Examining the number of surveys completed per day, per enumerator – helps inform your progress towards the daily targets and ultimately the survey target (a factor that has a direct impact on your survey budget) as well as can be used to flag enumerators dillydallying on their work.

(With such analysis, you can easily monitor the performance of each enumerator – adjust where need be and ensure survey resources are well managed and utilized – avoid overspending).
7. Number of outliers
Outliers are data points which differ significantly from other observations. These should be checked and tracked for each enumerator. Enumerators with high number of outliers might need to be retrained or might be an indication of data fraud – which can further be cross-checked and addressed.
8. Examine open-end responses
For surveys with open-end questions, it’s necessary to examine the open-end responses and other ‘other specify’ responses for gibberish or excessively vague answers.
9. Flat-lining check
For surveys with questions requiring rating, participants might select same answer for all, forming a perfect flatline (a metric which could indicate fatigue or enumerators’ attempt to fabricate the data).
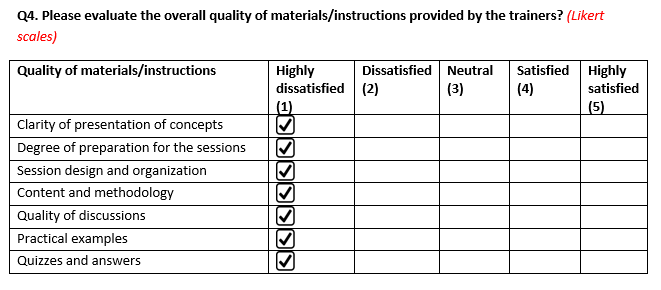
(Such anomalies should be tracked and flagged for further cross-checking)
10. Red-herrings check
Red herring is a type of survey question which includes fake answer among a set of valid answers. Such questions can be tracked and used to flag respondents or enumerators fabricating data.
For example, a question may ask: what is your most preferred analytics software? The answer choices may include R, Python, Excel, Tableau, PowerBI, and Printer. Obviously, the last choice is neither a software nor an analytics tool, and therefore you can track such responses and flag them for further cross-checking.
Track all metrics in one place! (Use an interactive dashboard)
Dashboard is a graphical user interface which provides at a-glance views of the Key Performance Indicators (KPIs) relevant to a particular business objective. In this case, a dashboard would help you track all the ten metrics explained above (in a single view) – enabling users (in this case the supervisors or the leadership) instantly spot metrics flagged for further checks and quickly address data issues before they are out of hand.
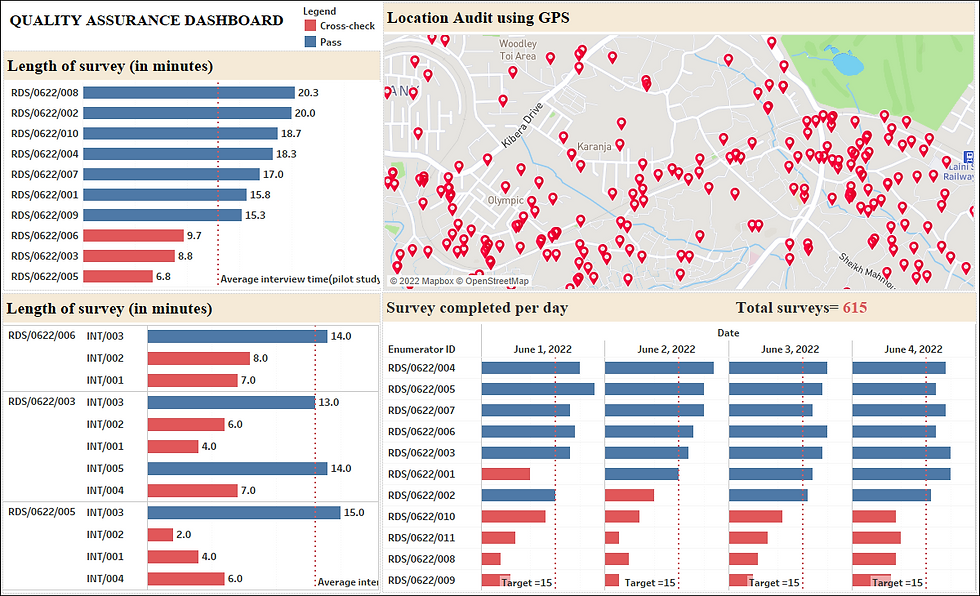
(Sample dashboard – showing you how you can combine different metrics to create a single view where you can track all the metrics at ago.)
Conclusion
These are some of the metrics you can include in your data quality assurance dashboard. Additional quality checks can always be included depending on the context of your survey.
Note the ability to detect data fraud largely depends on the rules you formulate with the data provider or the data team.
If you like the work we do and would like to work with us, drop us an email on our Contacts page and we’ll reach out!
Thank you for reading!